How we roll
We´re Narrative
We´re not claiming to reinvent the wheel in AI-driven content creation, but rather, we´re focusing on a specific, crucial aspect: producing effective SEO and affiliate texts. And doing this at scale, in a predictable, repeatable way.
The challenge that we addresses is both simple and complex: creating content that resonates with both search engines and real people. It's a task that requires not just understanding algorithms but also human interests and preferences. This is where our use of Retrieval-Augmented Generation (RAG) and fine-tuning comes into play. These techniques aren't about making grand statements; they're about adding subtlety and depth to AI capabilities, enabling it to produce more relevant and engaging content. Let´s start at the beginning - what is RAG and fine tuning?
The Basics of RAG and Fine-Tuning in AI Text Generation
RAG works by actively pulling in data from various external sources, ensuring that the content remains up-to-date and dynamic. This approach is particularly useful in environments where information changes rapidly, allowing the AI to offer the freshest insights. On the other hand, fine-tuning is more about refining the AI's existing knowledge base, aligning it more closely with specific writing styles, tones, or domain-specific nuances. It's like giving the AI a crash course in what makes great content for a particular niche.
While RAG excels in leveraging external knowledge and adapting to evolving data landscapes, fine-tuning enables the AI to align more deeply with particular writing styles or domain-specific vocabularies. Both these techniques, when used together, offer a comprehensive solution to the challenges of creating engaging and relevant content.
Combining RAG and Fine-Tuning for Optimal Performance
The magic happens when we synergize RAG and fine-tuning. This combination allows us to harness the strengths of both techniques, offering a more robust solution for our content generation needs. RAG's ability to pull in updated, external information complements fine-tuning's knack for precision and relevance, creating a balance between up-to-date information and stylistic alignment.
This synergy is particularly beneficial in reducing errors and hallucinations, common pitfalls in AI-generated content. RAG's reliance on external data sources provides a grounding effect, reducing the likelihood of fabrications, while fine-tuning helps the model stay true to the domain-specific context, further minimizing inaccuracies.
Moreover, this approach aligns well with our commitment to transparency and user trust. RAG's process of breaking down response generation into distinct stages offers insights into how the content is developed, fostering trust in the outputs. Meanwhile, fine-tuning ensures that the content aligns with the specific requirements and limitations of our clients' projects, delivering a tailored experience that resonates with their audience.
In summary, by combining RAG and fine-tuning, we at Narrative are not just throwing AI jargon around but are actively employing these techniques to deliver content that is both relevant and reliable. This is our way of embracing the complexities of AI-driven content generation, ensuring that our outputs are not just data-driven but also finely tuned to the nuances of human interest and engagement.
Discover New Blog Posts
Stay updated with our latest blog content.
A Dance of Words and Wires
.png)
What does Narrative offer?
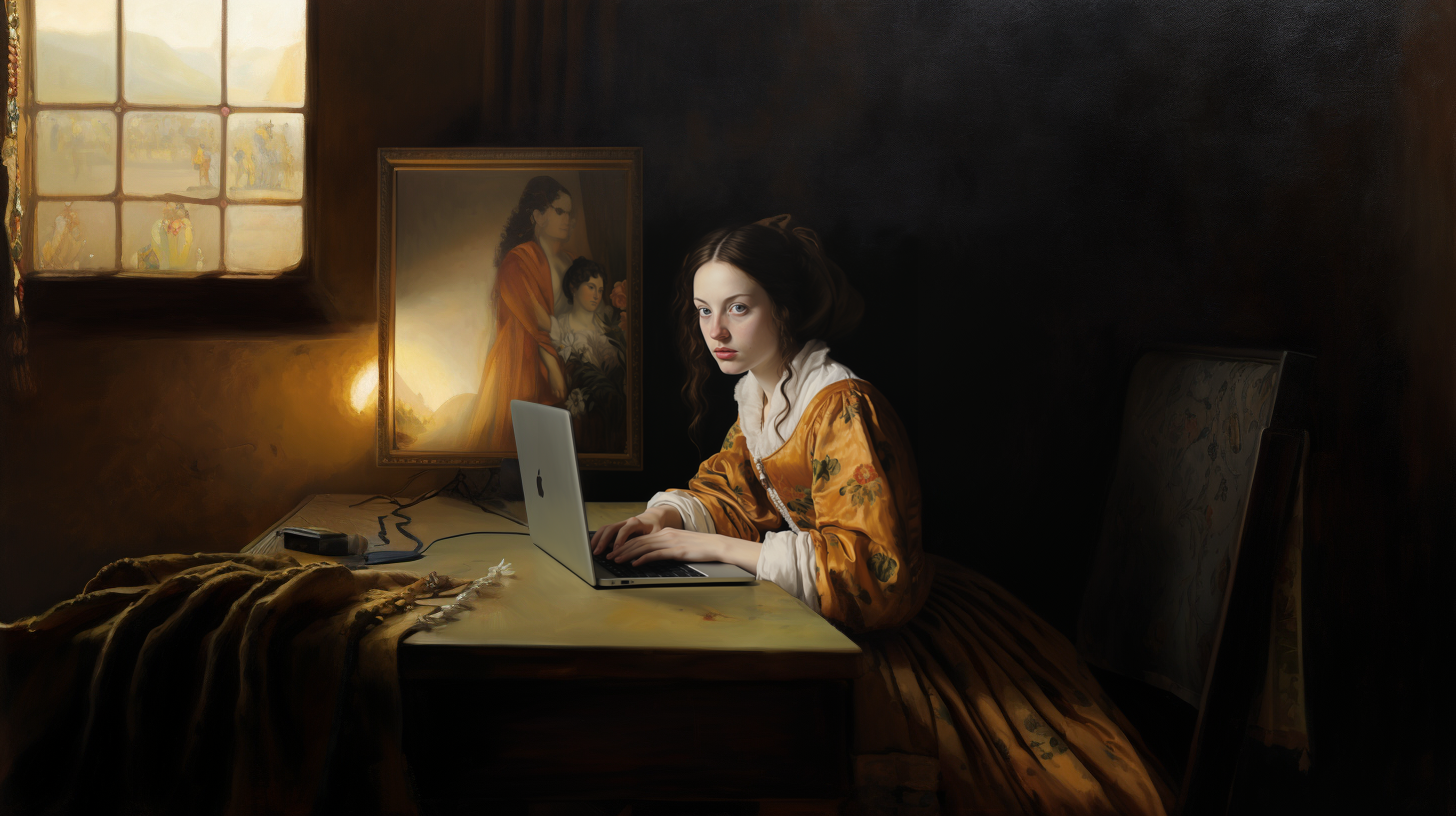