A Dance of Words and Wires
The Casino Conundrum
In an era where artificial intelligence soon blends into the tapestry of daily life, from curating personal shopping experiences to powering sophisticated virtual assistants, the advent of Large Language Models (LLMs) like GPT-4 stands as a testament to the extraordinary strides in technology.
These advanced AI systems, capable of generating strikingly human-like text, have not just captured the imagination of the tech world but have also become pivotal tools across diverse sectors.
Yet, beneath the surface of this technological marvel lies an intriguing conundrum.
While LLMs have been lauded for their eloquence and versatility, certain scenarios reveal a peculiar anomaly in their output. We, at Narrative noticed this whilst using these models to craft affiliate articles for online gaming sites. Here, instead of the polished prose one might expect, the language generated often degrades into a jarring and incomprehensible mishmash. This unexpected twist in the AI's linguistic abilities poses a compelling question: Could the quality of training data, drawn from the vast and variable expanse of the internet, be responsible for these linguistic hiccups?
Short answer: yes.
The Marvel of Large Language Models
At the core of LLMs is a concept known as machine learning, where the AI is 'trained' using enormous datasets. These datasets are often gleaned from the internet, encompassing a vast array of text that includes literature, news articles, online discussions, and more. This extensive training enables the AI to recognize patterns, nuances, and the intricacies of language, much like a child learning to speak by listening to the conversations around them.
The capabilities of these models are nothing short of remarkable. They can write essays, create poetry, generate technical reports, and even engage in witty banter. Their applications extend far beyond these creative pursuits, infiltrating sectors like customer service, where they power sophisticated chatbots, and education, where they assist in creating personalized learning materials.
One of the most striking aspects of LLMs is their ability to mimic different writing styles. Whether it's emulating the prose of a 19th-century novelist, drafting a legal document, or composing an informal blog post, these models adjust their tone and style with an ease that is almost human. This versatility is not just a technical achievement but a window into a future where AI can seamlessly integrate into various aspects of human life, enhancing and perhaps even transforming the way we communicate.
An Unanticipated Quirk
The proficiency of Large Language Models (LLMs) in generating coherent and contextually appropriate text is widely acknowledged. However, their application in creating content for online gaming affiliate articles unveils a peculiar anomaly: a noticeable decline in the quality of language. This deviation from the expected standard of eloquence and clarity is not just an oddity but a revealing insight into the underlying mechanics of these AI models.
The root of this issue lies in the training data used to develop these models. LLMs, such as GPT-4, are trained on vast datasets, predominantly sourced from the internet. This training process involves absorbing and analyzing a colossal amount of text, encompassing a wide spectrum of quality and style. In theory, this should enable the AI to generate well-rounded and versatile content. However, the internet, as a source, is replete with uneven and sometimes low-quality content, especially in niche areas like online gaming promotions. These segments are often dominated by SEO-driven articles, which prioritize keywords and search engine rankings over quality and readability.
When LLMs are prompted to generate content for such niches, they inadvertently draw on these subpar examples as part of their training. This results in outputs that mirror the flaws of their training material - the language becomes repetitive, stilted, or overly optimized for search engines, losing the natural flow and engagement quality of well-crafted writing. This phenomenon starkly contrasts with the AI's performance in other domains, where the training data is more diverse and of higher quality.
This unexpected outcome underscores a significant challenge in AI development: the dependency on available data. The quality of the output is intrinsically tied to the quality of the input. In domains where high-quality content is abundant, LLMs excel, showcasing their potential to mimic and even enhance human-like writing. But in areas flooded with poor-quality content, the AI struggles to rise above the limitations of its training materials.
The Root of the Problem – Training Data Challenges
This issue of declining language quality in affiliate articles for online gaming sites, created by Large Language Models (LLMs), underscores a fundamental challenge in AI development: the impact of training data. The performance of these models, including their ability to generate coherent and context-appropriate text, is deeply rooted in the nature and quality of the data they are trained on.
The training process of LLMs does not inherently distinguish between high and low-quality content; it assimilates and learns from all available data. As a result, when these models are prompted to generate content in domains dominated by subpar material, they tend to replicate similar patterns and styles. This phenomenon is a reflection of the 'garbage in, garbage out' principle in data science, where the quality of output is directly affected by the quality of input.
For users of LLMs, addressing this challenge is not straightforward. One approach that has shown promise involves looping articles through multiple rounds of short prompts. This technique aims to refine the model's output by iteratively guiding it towards better-quality content. Each loop acts as a retraining session, allowing the model to adjust its language generation based on the new input, gradually steering away from the low-quality patterns it learned initially.
While this method can mitigate the issue to some extent, it is not a panacea. It is labor-intensive and may not always yield consistently high-quality results. This challenge points to a larger, more complex problem in AI development: how to equip LLMs with the ability to discern and prioritize quality in their learning process. Solving this requires innovative approaches in AI training, possibly integrating more sophisticated content evaluation mechanisms and more selective data sourcing strategies.
A Dance of Words and Wires
In this intricate ballet of bytes and language, where Large Language Models pirouette across the digital stage, we find ourselves at an intriguing crossroads. As we teach these silicon-based scribes to mimic the nuances of human expression, we are reminded of the delicate balance between art and algorithm.
We're not just teaching AI to write better; we're engaging in a dialogue with our own creations, a conversation that spans the gap between the organic and the artificial. In this dance, we lead, but we also follow, learning as much about ourselves as we do about the capabilities and potential of the AI we've birthed.
At Narrative, our goal is clear: to consistently deliver high-quality texts that not only meet but exceed the expectations of our clients and users. Of course this means clients and users in the gaming and casino industries as well!
Understanding the dynamic nature of AI and language, our team is relentlessly working on refining the workflows and training processes of our apps and templates.
This involves not only leveraging the latest advancements in machine learning and natural language processing but also incorporating valuable feedback and insights from our users.
As we move forward, we at Narrative are excited about the possibilities and the future of AI in language generation. We are dedicated to being at the forefront of this field, continually pushing the boundaries to offer state-of-the-art solutions that redefine what is possible with AI-generated text.
Discover New Blog Posts
Stay updated with our latest blog content.
How we roll
.png)
What does Narrative offer?
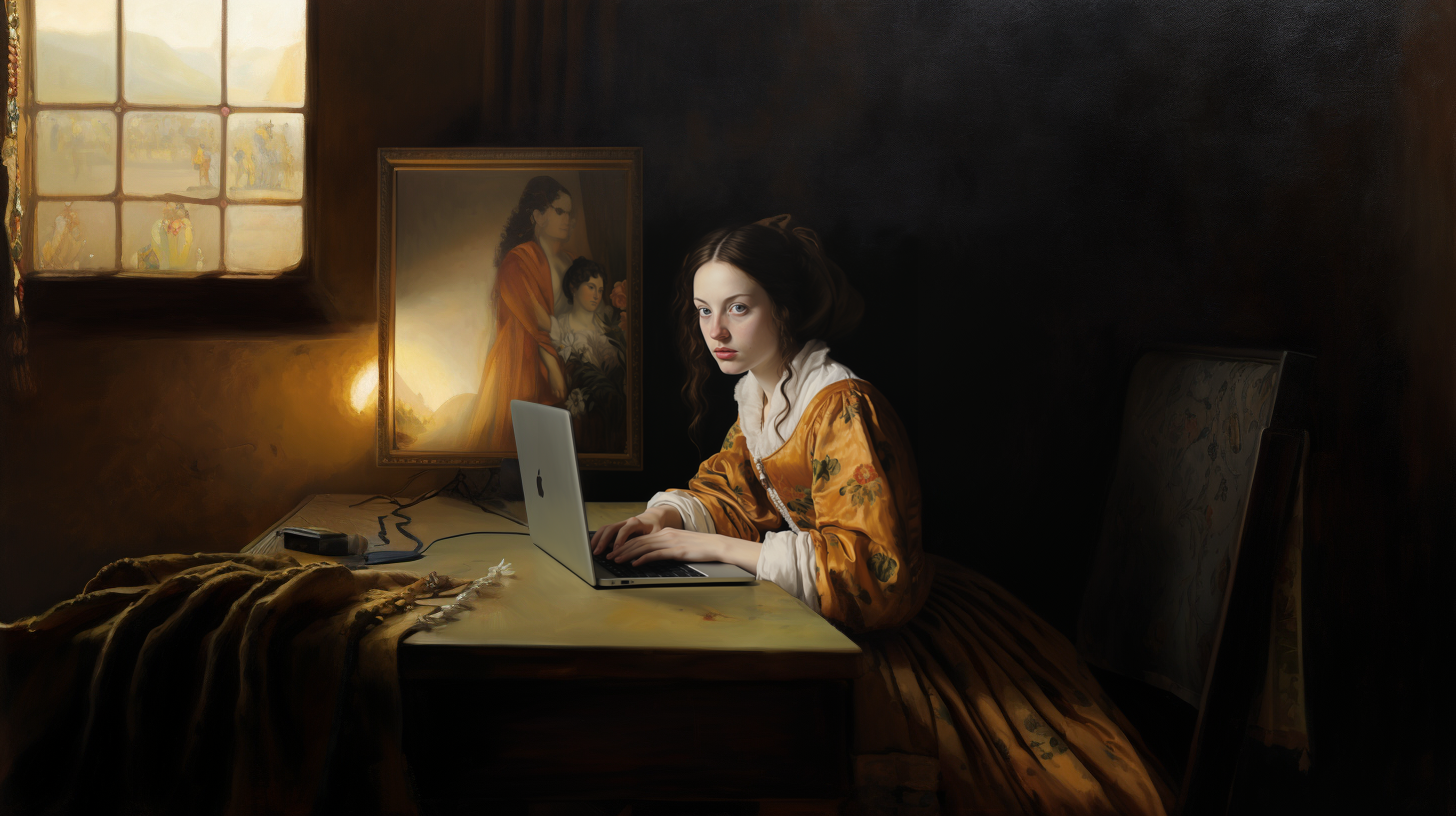